5/27/2022
BY: ANTHONY WELSH
By now, you should understand how we can take the mathematical formula Y=f(x) + N to create a data-driven culture of thinking. We’ve focused on the Y, the output, and the x, the input. Be sure to read the first three articles of this series to capture the whole picture.
Continuing with the right side of the equation, let’s talk about the N. If there is one thing that impedes our progress when we’re trying to learn, it’s noise. Noise is a fickle thing, and it can get in the way for new experimenters. Let’s spend this last article discussing strategies to deal with it.
CAPTURING THE NOISE
I like how Donald Wheeler, author and statistician, says “While all data contain noise, some data contain signals. Before you can detect a signal, you must filter out the noise.” I’ll expand this notion to include not only your data, but also how you collected that data in the first place.
What noise did you capture in the data? Perhaps more importantly, what noise did you not capture? Whether we like it or not, noise is always present. If you can, think back to your middle or high school physics classes where the teacher would have you assume a frictionless surface, no wind resistance, constant gravity, homogenous density, material properties are known, etc. They do this to keep things simple so you can learn the concepts. But, in the real world, variation is ever present, and it shows up in your data and affects your processes and products. It’s not good enough if things work in the lab or on some prototype. We need robust designs that work in the presence of real-life noise.
PAY ATTENTION TO THE NOISE
Some of the available strategies are applied after we have the data, but others we can apply in how we gather the data. The worst strategy of all, if you can even call it that, would be to consciously ignore the noise. This could look like testing a single prototype, changing settings of a machine based on a single output, believing your experiences are the only ones possible, not brainstorming with the team or testing only the “usual suspects” of ideas over and over. We must do better.
When designing an experiment, consider the factors you are manipulating and the things you are not manipulating. What do I mean? Think outside the box and pay attention to the possible background variables, the measurement system, sample sizes, statistical power, the opportunity for repeats, etc. Focus on what else could change between treatments besides the factors. The things you were trying to hold constant across treatments, ask yourself, were they constant? Surely the things we choose to manipulate in the experiment are not always a complete list of every active effect. Sometimes, the things we are not manipulating in the experiment turn out to be the most important learning that comes out of the study!
STRATEGIZE THE NOISE
There are many noise strategies to consider. In Six Sigma training, we go through the most applied strategies to help new practitioners avoid mistakes. I’ll provide a summary of five such strategies that are a good idea to use if you’re able.
-
Always validate your measurement system. To ensure there isn’t too much variation in your data gathering process, make sure you have a valid measurement system. Optimizing the measurement system and reducing the noise in your numbers can make your life easier while trying to learn about factors. During the measurement system analysis (MSA) you’ll also specifically quantify the measurement error, which can be useful.
-
Study a process that is in control. This means it exhibits a stable, predictable and consistent amount of random noise (variation). If the process is producing unpredictable variation, that makes it difficult to try any type of improvement efforts. Your best option would be to apply some standardization and root cause techniques to understand why the process is not stable. Solve that first, it’s your biggest signal, and then move on quantifying the Y=f(x).
-
Randomization is always a good idea. Let’s say there is background noise you are not aware of. Randomization takes this noise and applies it randomly to each observation, essentially spreading its effect equally across all your samples. That’s good because we wouldn’t want it to line up with any of your factor manipulation. The other benefit of randomization is in the analysis step because it helps fulfill some statistical assumptions. I concede randomization is not always possible, so if you can’t do it, you must account for that in the analysis to arrive at the correct conclusions. If randomization is not possible in your world, we cover how to do this in
Six Sigma Black Belt as well as our
Advanced DOE class.
-
Take copious notes. Someone once taught me our memory is not so reliable. Dig into the topic of memory and you’ll find things like hindsight bias, illusory correlation, self-serving bias, source amnesia, etc. Our memory is nothing like computer memory, so don’t rely on it. You should be taking copious amounts of notes during any study that can become a reference. Notice someone didn’t follow a procedure on a particular step? Write it down. Did someone drop one of the parts at some point? Write it down. While completing the analysis use those notes to provide real evidence instead of relying on your memory.
-
Repeats. If you can take repeats of a treatment without much extra cost, always capitalize on that option. Repeats, as a strategy, allow you to increase your sample size of a given treatment. Larger sample sizes help you get an average measurement which better matches what the underlying ‘truth’ should have been. By now you know any given data point is not perfect. The observed effect is really the true effect plus some amount of noise. To prove that, take a second measurement and I bet it’ll be different than the first. If it's not, you probably have a discrimination problem, but we should have already fixed that with strategy one. Each observation is subject to noise, and as such, isn’t going to be the perfect answer. On average, many individual observations can begin to estimate the truth very well. The number of repeats depends on the context, but there is usually a good starting point.
THE 4-AXIS OF NOISE
To navigate noise strategies, we explore what’s called the 4-Axis of Noise in our Six Sigma class. We start with determining the noise source and then rate it based on the 4-Axis of Noise - our ability to measure it, whether we can control it, how much knowledge we have of it, and finally, its frequency of change. Thinking along these axes we can determine which remaining noise strategies might be helpful.
SIX SIGMA AT THE CENTER
The Center’s courses combine proven problem-solving methods with powerful statistical tools to construct a reliable roadmap to improve business operations. Learn more about the Six Sigma courses we offer
here.
MEET OUR EXPERT:
Anthony Welsh, Six Sigma Master Black Belt
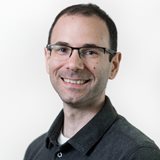
Anthony Welsh is a Six Sigma Master Black Belt with 20 years of experience delivering projects to both the automotive and consumer products industries. In his role at The Center, Anthony shares expert tools in critical thinking and data-driven decision making to assist clients with using Six Sigma methods to achieve real results.
Since 1991, the Michigan Manufacturing Technology Center has assisted Michigan’s small and medium-sized businesses to successfully compete and grow. Through personalized services designed to meet the needs of clients, we develop more effective business leaders, drive product and process innovation, promote company-wide operational excellence and foster creative strategies for business growth and greater profitability. Find us at www.the-center.org.
Categories: Leadership/Culture,
Six Sigma,
The Center